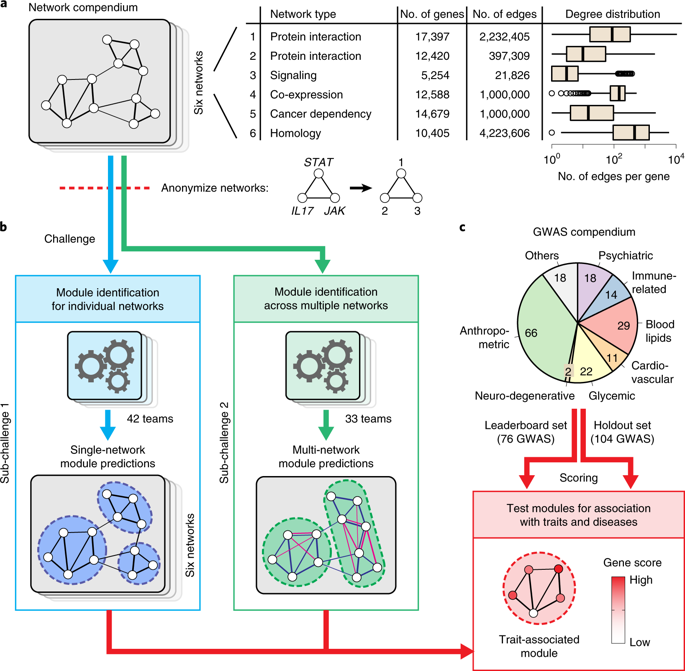
The outcome of the DREAM Challenge on Disease Module Identification in genetic networks has been reported in a paper published in Nature Methods. Over 400 participants from all around the world have contributed 75 different clustering algorithms to predict disease-relevant modules in diverse gene and protein networks. Participants could only use unsupervised clustering algorithms, which rely exclusively on the network structure and do not depend on additional biological information such as known disease genes. CNetS professor Santo Fortunato and former postdoc Lucas Jeub participated in the analysis of the results delivered by the algorithms.
The evaluation of predicted modules is challenging because there is no ground truth of ‘correct’ modules in molecular networks. Therefore a framework was introduced to empirically assess modules based on their association with complex traits and diseases using genome-wide association studies (GWAS) data. Kernel clustering, modularity optimization, random-walk-based and local methods were among the top performers, suggesting that no single type of approach is inherently superior and that multiple techniques should be used to identify complementary modules.
This work provides important guidelines on how to perform cluster analysis in molecular networks.