The aim of this project is to characterize, study and model various sources of bias that emerge from the complex network structure of the Web, social media, and search engines. Some of the questions we’re currently exploring concern how social, cognitive, and algorithmic biases lead to the emergence of information overload and online echo chambers that make us more vulnerable to abuse and manipulation.
Social and cognitive biases
Social media have become a prevalent channel to access information, spread ideas, and influence opinions. However, it has been suggested that social and algorithmic filtering may cause exposure to less diverse points of view. In the paper Measuring Online Social Bubbles we quantitatively measure this kind of social bias at the collective level by mining a massive datasets of web clicks. Our analysis shows that collectively, people access information from a significantly narrower spectrum of sources through social media and email, compared to a search baseline. The significance of this finding for individual exposure is revealed by investigating the relationship between the diversity of information sources experienced by users at both the collective and individual levels in two datasets where individual users can be analyzed—Twitter posts and search logs. There is a strong correlation between collective and individual diversity, supporting the notion that when we use social media we find ourselves inside “social bubbles.” Our results could lead to a deeper understanding of how technology biases our exposure to new information. A release about this work got some press coverage and an extended version of this paper is in preparation.
We also find that the combination of social media mechanisms and cognitive biases such as limited attention and information overload may explain the viral spread of low-quality information, such as the digital misinformation that threatens our democracy. We develop a stylized model of an online social network, where individual agents prefer quality information, but have behavioral limitations in managing a heavy flow of information. The model predicts that in realistic conditions, low-quality information is just as likely to go viral, providing an interpretation for the high volume of misinformation we observe online.
Gender bias
Contributing to the writing of history has never been as easy as it is today. Anyone with access to the Web is able to play a part on Wikipedia, an open and free encyclopedia, and arguably one of the primary sources of knowledge on the Web. In our paper First Women, Second Sex: Gender Bias in Wikipedia we study gender bias in Wikipedia in terms of how women and men are characterized in their biographies. To do so, we analyze biographical content in three aspects: meta-data, language, and network structure. Our results show that, indeed, there are differences in characterization and structure. Some of these differences are reflected from the off-line world documented by Wikipedia, but other differences can be attributed to gender bias in Wikipedia content. We contextualize these differences in social theory and discuss their implications for Wikipedia policy. This work was covered in Wikimedia Research Newsletter. An extended journal version titled Women through the glass ceiling: gender asymmetries in Wikipedia also shows that women in Wikipedia are more notable than men, which we interpret as the outcome of a subtle glass ceiling effect.
Popularity bias
The feedback loops between users searching information, users creating content, and the ranking algorithms of search engines that mediate between them, lead to surprising results. We are studying how all these systems and communities influence and feed on each other in a dynamic information ecology, and how these interactions affect their evolution and their impact on the global processes of information discovery, retrieval, and utilization.
For example, studying the relationship between Web traffic and PageRank, we have shown that given the heterogeneity of topical interests expressed by search queries, search engines mitigate the popularity bias generated by the rich-get-richer structure of the Web graph. These results, dispelling the feared Googlearchy affect, have been published in Proc. Natl. Acad. Sci. USA, presented at the WAW 2006 keynote (slides), and generated some media attention. You can see some movies demonstrating the finding. The result also inspired a robust rank-based model of scale-free network growth, published in Phys. Rev. Lett. (press release).
Most recently we have identified the conditions in which popularity may be a viable proxy for quality content by studying a simple model of cultural market endowed with an intrinsic notion of quality. A parameter representing the cognitive cost of exploration controls the critical trade-off between quality and popularity. There is a regime of intermediate exploration cost where an optimal balance exists, such that choosing what is popular actually promotes high-quality items to the top. Outside of these limits, however, popularity bias is more likely to hinder quality.
Censorship
We also studied sources of bias that stem from legal, political, or economic factors. The CENSEARCHIP tool visualizes the differences between results obtained from different search engines, or different country versions of a search engine. This tool, based on a technique described in this paper in First Monday, generated a lot of reactions in the media and the blogosphere (press release).
Project Contributors



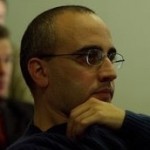
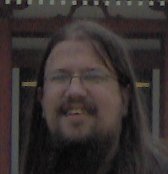
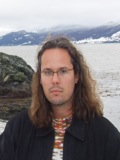
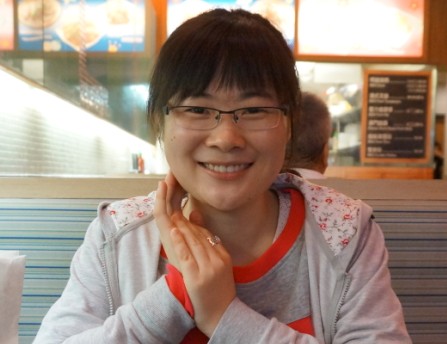

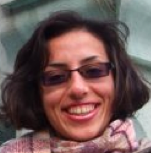


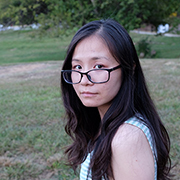
Support
Mark Meiss was supported by the Advanced Network Management Laboratory, one of the Pervasive Technology Labs established at Indiana University with funding from the Lilly Endowment.
Santo Fortunato was supported by a Volkswagen Foundation grant.
Diego Fregolente was supported by the J.S. McDonnell Foundation.
This research was also supported in part by the National Science Foundation under awards 0348940, 0513650, and 0705676.
Opinions, findings, conclusions, recommendations or points of view of this group are those of the authors and do not necessarily represent the official position of the National Science Foundation, the Volkswagen Foundation, the McDonnell Foundation, or Indiana University.